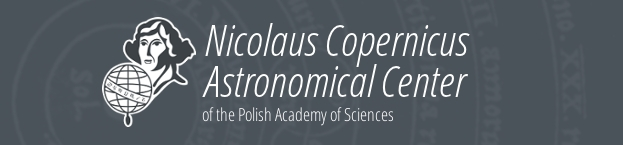
Degree: Ph.D.
Position: Post-Doc
Division: Astrophysics II (Warsaw)ORCID: 0000-0003-0594-9138
Office: 103
Phone: +48 223296103
E-mail: ghajdu@camk.edu.pl
Gergely Hajdu is a postdoctoral researcher at the Nicolaus Copernicus Astronomical Center (CAMK) in Warsaw where he is a member of the international Araucaria team working on the cosmic distance scale.
During his doctoral research, he studied the the near-infrared light-curve properties of RR Lyrae variables, their distribution in the Galactic disk, as well as the distribution of Classical Cepheid variables in the obscured Galactic disk using data from the VISTA Variables in the Via Lactea survey. He obtained a joint double degree from the Instituto de Astrofísica of the Pontificia Universidad Católica de Chile (Santiago, Chile), and the Astronomisches Rechen-Institut of the Ruprecht-Karls-Universität (Heidelberg, Germany) in 2013. Before his PhD studies, he worked as a research assistant at the Konkoly Observatory of the Hungarian Academy of Sciences, Budapest, Hungary (2010-2013).
Dr. Hajdu has wide experience in observational astronomy, including obtaining and reducing optical and near-infrared observations, and data analysis using modern techniques (including the construction and deployment of machine learning models).
In 2019 he moved to Poland, joining the Araucaria group as a postdoctoral researcher. Besides working towards the main goal of the group of improving distance determination methods, he is especially interested in the detection and characterization of binary RR Lyrae systems. Another focus of his attention is the application of machine learning methods to astronomical problems, such as the development of light-curves shape – physical parameter relationships for Classical Cepheids and RR Lyrae variables.
His publications listed by the NASA ADS service can be found here.