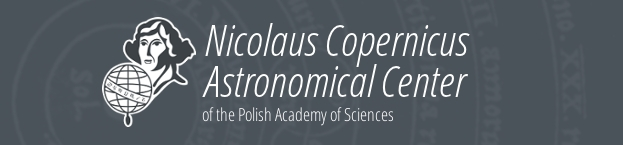
Sudhagar Suyamprakasam (NCAC, Warsaw)
Most all-sky searches for continuous gravitational waves assume the source to be isolated. In this paper, we allow for an unknown companion object in a long-period orbit and opportunistically use previous results from an all-sky search for isolated sources to constrain the continuous gravitational-wave amplitude over a large and unexplored range of binary orbital parameters without explicitly performing a dedicated search for binary systems. The resulting limits are significantly more constraining than any existing upper limits for unknown binary systems, albeit the latter apply to different orbital parameter ranges that are computationally much costlier to explore.
Sudhagar Suyamprakasam (NCAC, Warsaw)
Despite achieving sensitivities capable of detecting the extremely small amplitude of gravitational waves (GWs), LIGO and Virgo detector data contain frequent bursts of non-Gaussian transient noise, commonly known as 'glitches'. Glitches come in various time-frequency morphologies, and they are particularly challenging when they mimic the form of real GWs. Given the higher expected event rate in the next observing run (O4), LIGO-Virgo GW event candidate validation will require increased levels of automation. Gravity Spy, a machine learning tool that successfully classified common types of LIGO and Virgo glitches in previous observing runs, has the potential to be restructured as a signal-vs-glitch classifier to accurately distinguish between glitches and GW signals. A signal-vs-glitch classifier used for automation must be robust and compatible with a broad array of background noise, new sources of glitches, and the likely occurrence of overlapping glitches and GWs. We present GSpyNetTree, the Gravity Spy Convolutional Neural Network Decision Tree: a multi-CNN classifier using CNNs in a decision tree sorted via total GW candidate mass tested under these realistic O4-era scenarios.
Helena Ciechowska (Institute of Geophysics, PAN, Warsaw)
Man-made changes to the environment can trigger seismic events. The Castanhao region (Ceara, Brazil) is an example of such activity. The dam built over the Jaguaribe River was followed by the creation of the Castanhao reservoir, which soon before the opening of the dam started to impact the occurrence of earthquake swarms in the area. In my work, I analyze the continuous data recorded on a seismic network of 6 seismological stations, between January 2010 and December 2010. In order to process such a vast dataset, the PyMPA template matching software is used along with STA/LTA algorithm.